Welcome
diffusers/ controlnet-depth-sdxl-1.0
API Sample: diffusers/controlnet-depth-sdxl-1.0
You don't have any projects yet. To be able to use our api service effectively, please sign in/up and create a project.
Get your api keyPrepare Authentication Signature
//Sign up Wiro dashboard and create project
export YOUR_API_KEY="{{useSelectedProjectAPIKey}}";
export YOUR_API_SECRET="XXXXXXXXX";
//unix time or any random integer value
export NONCE=$(date +%s);
//hmac-SHA256 (YOUR_API_SECRET+Nonce) with YOUR_API_KEY
export SIGNATURE="$(echo -n "${YOUR_API_SECRET}${NONCE}" | openssl dgst -sha256 -hmac "${YOUR_API_KEY}")";
Create a New Folder - Make HTTP Post Request
Create a New Folder - Response
Upload a File to the Folder - Make HTTP Post Request
Upload a File to the Folder - Response
Run Command - Make HTTP Post Request
curl -X POST "{{apiUrl}}/Run/{{toolSlugOwner}}/{{toolSlugProject}}" \
-H "Content-Type: {{contentType}}" \
-H "x-api-key: ${YOUR_API_KEY}" \
-H "x-nonce: ${NONCE}" \
-H "x-signature: ${SIGNATURE}" \
-d '{{toolParameters}}';
Run Command - Response
//response body
{
"errors": [],
"taskid": "2221",
"socketaccesstoken": "eDcCm5yyUfIvMFspTwww49OUfgXkQt",
"result": true
}
Get Task Detail - Make HTTP Post Request
curl -X POST "{{apiUrl}}/Task/Detail" \
-H "Content-Type: {{contentType}}" \
-H "x-api-key: ${YOUR_API_KEY}" \
-H "x-nonce: ${NONCE}" \
-H "x-signature: ${SIGNATURE}" \
-d '{
"tasktoken": 'eDcCm5yyUfIvMFspTwww49OUfgXkQt',
}';
Get Task Detail - Response
//response body
{
"total": "1",
"errors": [],
"tasklist": [
{
"id": "2221",
"uuid": "15bce51f-442f-4f44-a71d-13c6374a62bd",
"name": "",
"socketaccesstoken": "eDcCm5yyUfIvMFspTwww49OUfgXkQt",
"parameters": {
"inputImage": "https://api.wiro.ai/v1/File/mCmUXgZLG1FNjjjwmbtPFr2LVJA112/inputImage-6060136.png"
},
"debugoutput": "",
"debugerror": "",
"starttime": "1734513809",
"endtime": "1734513813",
"elapsedseconds": "6.0000",
"status": "task_postprocess_end",
"cps": "0.000585000000",
"totalcost": "0.003510000000",
"guestid": null,
"projectid": "699",
"modelid": "598",
"description": "",
"basemodelid": "0",
"runtype": "model",
"modelfolderid": "",
"modelfileid": "",
"callbackurl": "",
"marketplaceid": null,
"createtime": "1734513807",
"canceltime": "0",
"assigntime": "1734513807",
"accepttime": "1734513807",
"preprocessstarttime": "1734513807",
"preprocessendtime": "1734513807",
"postprocessstarttime": "1734513813",
"postprocessendtime": "1734513814",
"pexit": "0",
"categories": "["tool","image-to-image","quick-showcase","compare-landscape"]",
"outputs": [
{
"id": "6bc392c93856dfce3a7d1b4261e15af3",
"name": "0.png",
"contenttype": "image/png",
"parentid": "6c1833f39da71e6175bf292b18779baf",
"uuid": "15bce51f-442f-4f44-a71d-13c6374a62bd",
"size": "202472",
"addedtime": "1734513812",
"modifiedtime": "1734513812",
"accesskey": "dFKlMApaSgMeHKsJyaDeKrefcHahUK",
"foldercount": "0",
"filecount": "0",
"ispublic": 0,
"expiretime": null,
"url": "https://cdn1.wiro.ai/6a6af820-c5050aee-40bd7b83-a2e186c6-7f61f7da-3894e49c-fc0eeb66-9b500fe2/0.png"
}
],
"size": "202472"
}
],
"result": true
}
Get Task Process Information and Results with Socket Connection
<script type="text/javascript">
window.addEventListener('load',function() {
//Get socketAccessToken from task run response
var SocketAccessToken = 'eDcCm5yyUfIvMFspTwww49OUfgXkQt';
WebSocketConnect(SocketAccessToken);
});
//Connect socket with connection id and register task socket token
async function WebSocketConnect(accessTokenFromAPI) {
if ("WebSocket" in window) {
var ws = new WebSocket("wss://socket.wiro.ai/v1");
ws.onopen = function() {
//Register task socket token which has been obtained from task run API response
ws.send('{"type": "task_info", "tasktoken": "' + accessTokenFromAPI + '"}');
};
ws.onmessage = function (evt) {
var msg = evt.data;
try {
var debugHtml = document.getElementById('debug');
debugHtml.innerHTML = debugHtml.innerHTML + "\n" + msg;
var msgJSON = JSON.parse(msg);
console.log('msgJSON: ', msgJSON);
if(msgJSON.type != undefined)
{
console.log('msgJSON.target: ',msgJSON.target);
switch(msgJSON.type) {
case 'task_queue':
console.log('Your task has been waiting in the queue.');
break;
case 'task_accept':
console.log('Your task has been accepted by the worker.');
break;
case 'task_preprocess_start':
console.log('Your task preprocess has been started.');
break;
case 'task_preprocess_end':
console.log('Your task preprocess has been ended.');
break;
case 'task_assign':
console.log('Your task has been assigned GPU and waiting in the queue.');
break;
case 'task_start':
console.log('Your task has been started.');
break;
case 'task_output':
console.log('Your task has been started and printing output log.');
console.log('Log: ', msgJSON.message);
break;
case 'task_error':
console.log('Your task has been started and printing error log.');
console.log('Log: ', msgJSON.message);
break;
case 'task_output_full':
console.log('Your task has been completed and printing full output log.');
break;
case 'task_error_full':
console.log('Your task has been completed and printing full error log.');
break;
case 'task_end':
console.log('Your task has been completed.');
break;
case 'task_postprocess_start':
console.log('Your task postprocess has been started.');
break;
case 'task_postprocess_end':
console.log('Your task postprocess has been completed.');
console.log('Outputs: ', msgJSON.message);
//output files will add ui
msgJSON.message.forEach(function(currentValue, index, arr){
console.log(currentValue);
var filesHtml = document.getElementById('files');
filesHtml.innerHTML = filesHtml.innerHTML + '<img src="' + currentValue.url + '" style="height:300px;">'
});
break;
}
}
} catch (e) {
console.log('e: ', e);
console.log('msg: ', msg);
}
};
ws.onclose = function() {
alert("Connection is closed...");
};
} else {
alert("WebSocket NOT supported by your Browser!");
}
}
</script>
Prepare UI Elements Inside Body Tag
<div id="files"></div>
<pre id="debug"></pre>
SDXL-controlnet: Depth
These are controlnet weights trained on stabilityai/stable-diffusion-xl-base-1.0 with depth conditioning. You can find some example images in the following.
prompt: spiderman lecture, photorealistic
Usage
Make sure to first install the libraries:
pip install accelerate transformers safetensors diffusers
And then we're ready to go:
import torch
import numpy as np
from PIL import Image
from transformers import DPTFeatureExtractor, DPTForDepthEstimation
from diffusers import ControlNetModel, StableDiffusionXLControlNetPipeline, AutoencoderKL
from diffusers.utils import load_image
depth_estimator = DPTForDepthEstimation.from_pretrained("Intel/dpt-hybrid-midas").to("cuda")
feature_extractor = DPTFeatureExtractor.from_pretrained("Intel/dpt-hybrid-midas")
controlnet = ControlNetModel.from_pretrained(
"diffusers/controlnet-depth-sdxl-1.0",
variant="fp16",
use_safetensors=True,
torch_dtype=torch.float16,
).to("cuda")
vae = AutoencoderKL.from_pretrained("madebyollin/sdxl-vae-fp16-fix", torch_dtype=torch.float16).to("cuda")
pipe = StableDiffusionXLControlNetPipeline.from_pretrained(
"stabilityai/stable-diffusion-xl-base-1.0",
controlnet=controlnet,
vae=vae,
variant="fp16",
use_safetensors=True,
torch_dtype=torch.float16,
).to("cuda")
pipe.enable_model_cpu_offload()
def get_depth_map(image):
image = feature_extractor(images=image, return_tensors="pt").pixel_values.to("cuda")
with torch.no_grad(), torch.autocast("cuda"):
depth_map = depth_estimator(image).predicted_depth
depth_map = torch.nn.functional.interpolate(
depth_map.unsqueeze(1),
size=(1024, 1024),
mode="bicubic",
align_corners=False,
)
depth_min = torch.amin(depth_map, dim=[1, 2, 3], keepdim=True)
depth_max = torch.amax(depth_map, dim=[1, 2, 3], keepdim=True)
depth_map = (depth_map - depth_min) / (depth_max - depth_min)
image = torch.cat([depth_map] * 3, dim=1)
image = image.permute(0, 2, 3, 1).cpu().numpy()[0]
image = Image.fromarray((image * 255.0).clip(0, 255).astype(np.uint8))
return image
prompt = "stormtrooper lecture, photorealistic"
image = load_image("https://huggingface.co/lllyasviel/sd-controlnet-depth/resolve/main/images/stormtrooper.png")
controlnet_conditioning_scale = 0.5 # recommended for good generalization
depth_image = get_depth_map(image)
images = pipe(
prompt, image=depth_image, num_inference_steps=30, controlnet_conditioning_scale=controlnet_conditioning_scale,
).images
images[0]
images[0].save(f"stormtrooper.png")
To more details, check out the official documentation of StableDiffusionXLControlNetPipeline.
Training
Our training script was built on top of the official training script that we provide here.
Training data and Compute
The model is trained on 3M image-text pairs from LAION-Aesthetics V2. The model is trained for 700 GPU hours on 80GB A100 GPUs.
Batch size
Data parallel with a single gpu batch size of 8 for a total batch size of 256.
Hyper Parameters
Constant learning rate of 1e-5.
Mixed precision
fp16
Tools
View All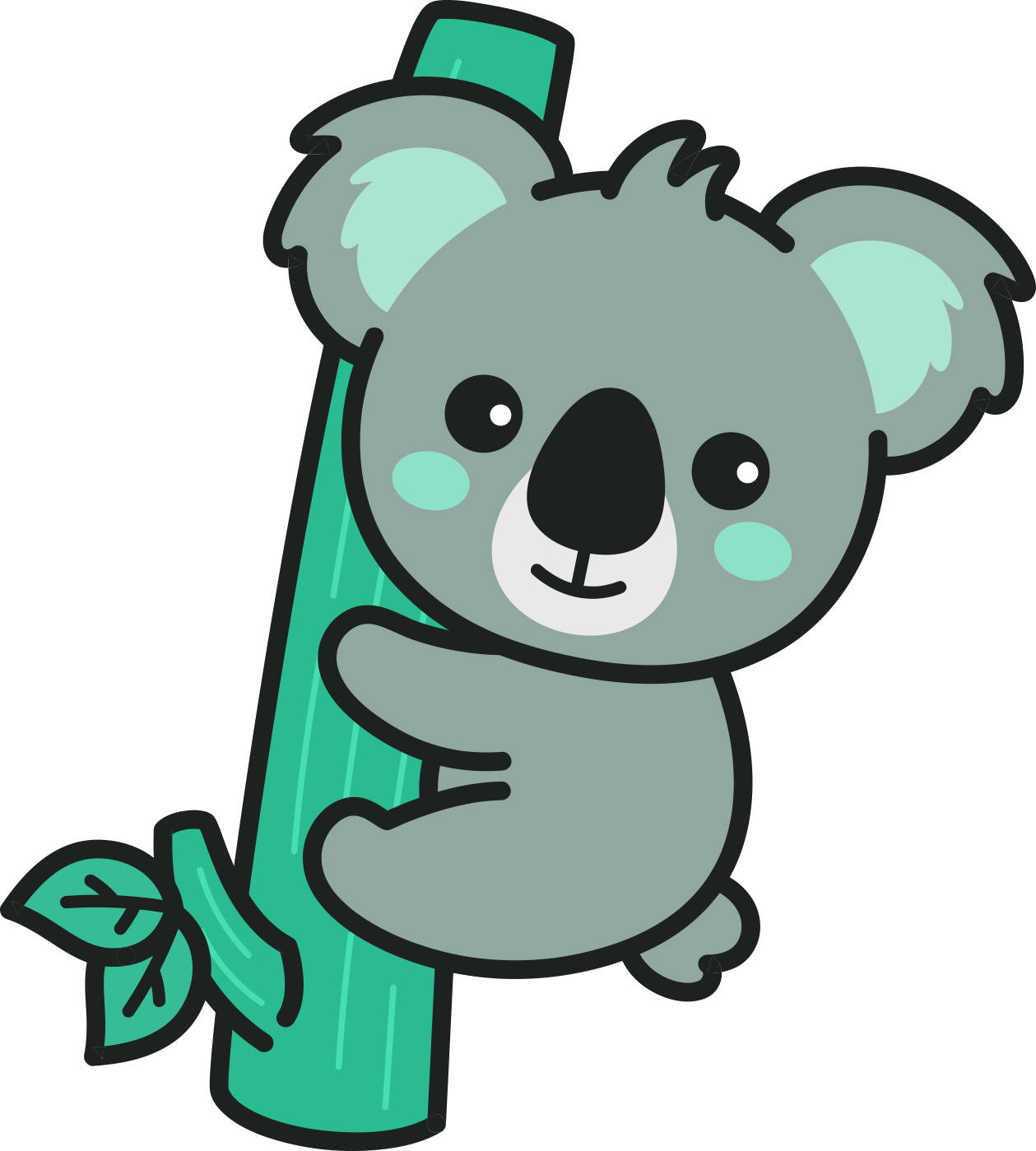
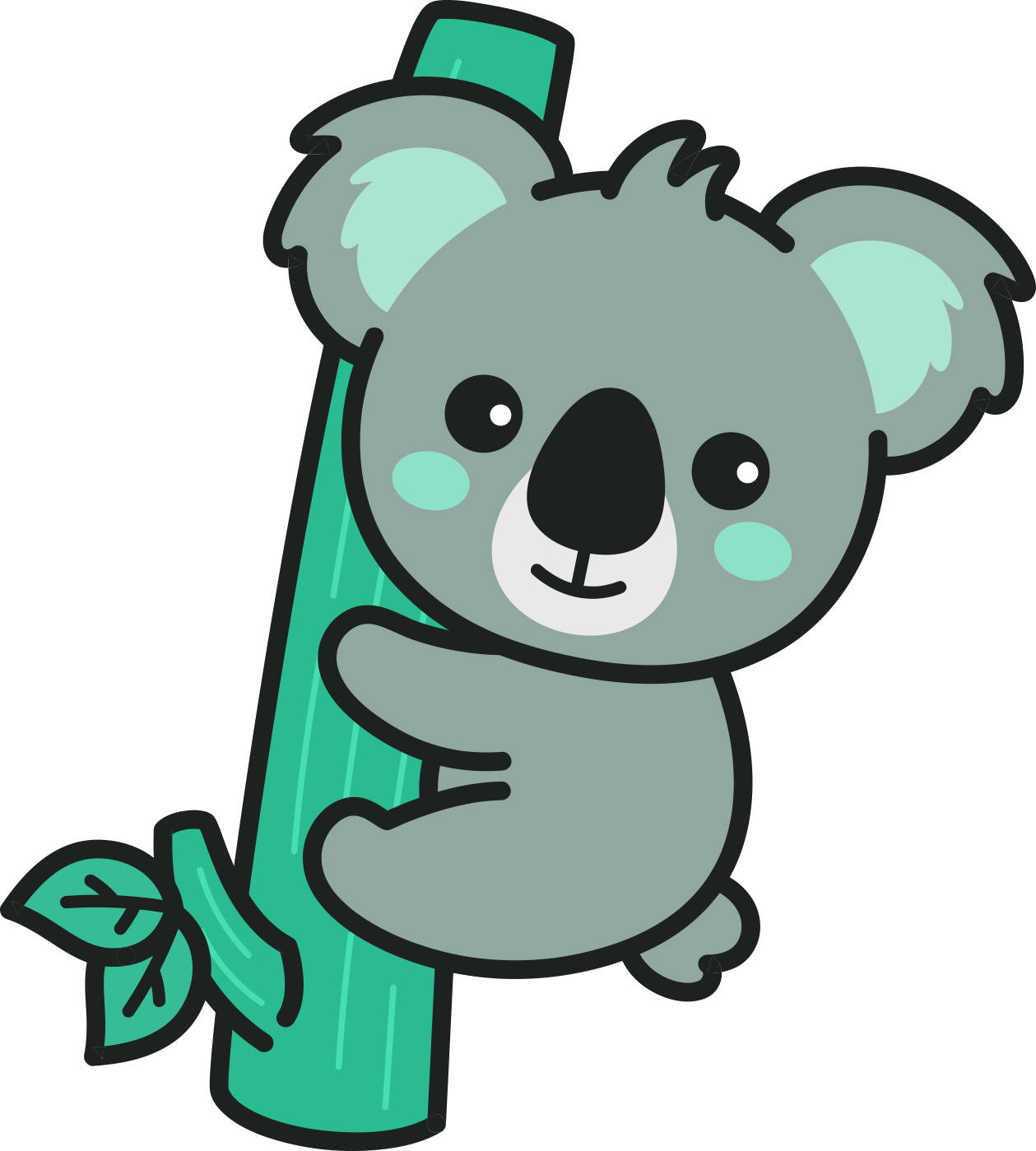